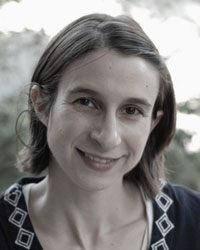
Cynthia Rudin, PhD
Associate Professor of Computer Science and Electrical and Computer Engineering
Duke University
Cynthia Rudin is an associate professor of computer science and electrical and computer engineering at Duke University, and directs the Prediction Analysis Lab. Her interests are in machine learning, data mining, applied statistics, and knowledge discovery (Big Data). Her application areas are in energy grid reliability, healthcare, and computational criminology. Previously, Prof. Rudin held positions at MIT, Columbia, and NYU. She holds an undergraduate degree from the University at Buffalo where she received the College of Arts and Sciences Outstanding Senior Award in Sciences and Mathematics, and three separate outstanding senior awards from the departments of physics, music, and mathematics. She received a PhD in applied and computational mathematics from Princeton University. She is the recipient of the 2013 and 2016 INFORMS Innovative Applications in Analytics Awards, an NSF CAREER award, was named as one of the “Top 40 Under 40” by Poets and Quants in 2015, and was named by Businessinsider.com as one of the 12 most impressive professors at MIT in 2015. Her work has been featured in Businessweek, The Wall Street Journal, the New York Times, the Boston Globe, the Times of London, Fox News (Fox & Friends), the Toronto Star, WIRED Science, U.S. News and World Report, Slashdot, CIO magazine, Boston Public Radio, and on the cover of IEEE Computer. She is presently the chair of the INFORMS Data Mining Section, and will be chair-elect of the Statistical Learning and Data Science section of the American Statistical Association.
Fulton Wang, Tyler McCormick, Cynthia Rudin, and John Gore. Modeling Recovery Curves With Application to Prostatectomy. ArXiv, 2014
Berk Ustun, Stefano Trac`a, and Cynthia Rudin. Supersparse Linear Integer Models for Predictive Scoring Systems, Proceedings of late breaking track, Twenty-Seventh AAAI Conference on Artificial Intelligence (AAAI-13), 2013.
Benjamin Letham, Cynthia Rudin, Tyler H. McCormick, and David Madigan. Building Interpretable Classifiers with Rules using Bayesian Analysis. In revision to Annals of Applied Statistics, 2013.
Benjamin Letham, Cynthia Rudin, Tyler McCormick, and David Madigan. Interpretable Classifiers using Rules and Bayesian Analysis: Building a Better Stroke Prediction Model, Proceedings of late breaking track, Twenty-Seventh AAAI Conference on Artificial Intelligence (AAAI-13), 2013.
Tyler McCormick, Cynthia Rudin, and David Madigan. Hierarchical Models for Association Rule Mining: A New Approach for Adverse Event Prediction in Clinical Trials, Annals of Applied Statistics, Volume 6, No. 2, pages 652–668, 2012.